Professor Martin Singull's research focuses on statistical inference of this type of data. In particular, the considered statistical models contain unknown parameters, which describe the expected values over time as well as all dependencies. The parameters need to be estimated so that the statistical models can be further used for prediction and classification of future unknown observations.
In his latest research projects, Martin Singull uses both the spatial and temporal information in repeated measurements, to develop efficient classifiers, and derive probabilities of misclassification, for future unknown units or individuals that are assumed to follow different expected values over time.
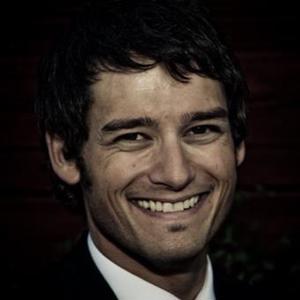
Martin Singull
Professor, Head of Division
Everywhere and all the time, huge amounts of data are generated that must be analyzed to be useful for the development of society. These data sets are large in that sense, that they may include many independent or dependent observations. They can also be more complex, with, for example, repeated measurements on the same units or individuals.
About me
Academic Degrees (Linköping University)
- Docent in Mathematical Statistics, March 2016
- Doctor of Philosophy PhD (Teknologie doktor), Mathematical Statistics, 2009
- Licentiate of Engineering (Teknologie licentiat), Mathematical Statistics, 2007
- Master of Science in Applied Physics and Electrical Engineering, 2003
Academic Experience (Linköping University)
- Head of Division – Applied Mathematics, 2021 -
- Professor in Mathematical Statistics, 2020
- Head of Division – Mathematical Statistics, 2016 - 2020
- Associate Professor in Mathematical Statistics, 2018
- Assistant Professor in Mathematical Statistics, 2012
Commissions of Trust
- Director for the Research School in Interdisciplinary Mathematics at Linköping University, 2024 -
- Expert group member (Technology) STINT, The Swedish Foundation for International Cooperation in Research and Higher Education, 2022 -
- Chair of the board of Cramérsällskapet (part of the Swedish Statistical Society), 2022 - 2024
- Team Leader for the Sida funded bilateral subprograms in mathematics and statistics at the five universities Royal University of Phnom Penh - Cambodia, Eduardo Mondlane University - Mozambique, University of Rwanda, University of Dar Es Salaam - Tanzania and Makerere University – Uganda (ended 2022)
- Member of ISP (International Science Programme) Mathematics Reference Group, 2021 -
- Board member of the Faculty of Science and Engineering, Linköping University, 2021-
- Member of the international scientific committee for the conference series International Workshop on Matrices and Statistics (IWMS), 2019 -
- Chair of organizing committee International Workshop on Matrices and Statistics (IWMS) at Linköping University, 2023
- Chair of organizing committee for the International Conference on Trends and Perspectives in Linear Statistical Inference (LinStat2014) at Linköping University, 2014
PhD students
Other PhD students
- Jean de Dieu Niyigena (University of Rwanda)
Project title: Approximation of misclassification probabilities using quadratic classifier for repeated measurements (main supervisor) - Moa Eriksson (BKV, Linköping University)
Preliminary project title: The impact of internal and external factors on the eye with a focus on retinal diseases (co-supervisor) - John Södling (HMV, Linköping University)
Preliminary project title: Ischemic Heart Disease, Socioeconomic Impact, and Prediction Models in Young Cancer Patients (co-supervisor)
Former PhD students
- Elias Erdtman (licentiate 2023)
Change point detection with respect to variance (main supervisor) - Emelyne Umunoza Gasana (doctoral 2023)
An Edgeworth-type Expansion of the Distribution of a Likelihood-based Classifier for Single Time-point Measurements and Growth Curves (main supervisor) - Denise Uwamariya (doctoral 2023)
Large deviations of condition numbers and extremal eigenvalues of random matrices (co-supervisor) - Béatrice Byukusenge (doctoral 2022)
Residual Analysis in the GMANOVA-MANOVA Model (main supervisor) - Pontus Söderbäck (doctoral 2022)
Decomposing the Option Pricing Problem: Estimating the Causal Factors: Interest Rates, Dividends, and Risk-Neutral Probabilities (co-supervisor) - Felix Wamono (doctoral 2021, Makerere University)
Contributions to reduced rank regression modelling with applications to small area estimation (co-supervisor) - Edward Kanuti Ngailo (2020, doctoral)
Contributions to linear discriminant analysis with applications to growth curves (main supervisor) - Andongwisye John Mwakisisile (licentiate 2018, doctoral UDSM 2018)
Asset Liability Management for Tanzania Pension Funds (co-supervisor) - Emanuel Evarest Sinkwembe (licentiate 2017, doctoral UDSM 2018)
Modelling Weather Dynamics for Weather Derivatives Pricing (main supervisor) - Innocent Ngaruye (doctoral 2017)
Contributions to Small Area Estimation: Using Random Effects Growth Curve Model (main supervisor) - Jolanta Pielaszkiewicz (doctoral 2015)
Contributions to High–Dimensional Analysis under Kolmogorov Condition (co-supervisor) - Joseph Nzabanita (doctoral 2015)
Bilinear and Trilinear Regression Models with Structured Covariance Matrices (co-supervisor)
Research
News
Publications
2025


2024



2023


2022

Books
Recent Developments in Multivariate and Random Matrix Analysis
Edited by Thomas Holgersson and Martin Singull
This volume is a tribute to Professor Dietrich von Rosen on the occasion of his 65th birthday. It contains a collection of twenty original papers. The contents of the papers evolve around multivariate analysis and random matrices with topics such as high-dimensional analysis, goodness-of-fit measures, variable selection and information criteria, inference of covariance structures, the Wishart distribution and growth curve models.
More about the book