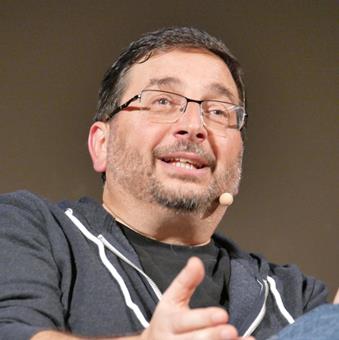
Hector Geffner
Visiting Professor
Founded the Representation, Learning and Planning lab.
Representation Learning for Acting and Planning
Hector Geffner is a Guest Wallenberg Professor at Department of Computer and Information Science (IDA) within the Artificial Intelligence and Integrated Computer Systems (AIICS), where he founded the Representation, Learning and Planning lab.
Recent progress in Artificial Intelligence (AI) has been remarkable due to developments in deep learning. Yet AI systems based on deep learning are not reliable. A good metaphor for understanding this limitation is provided by the account of human thinking pioneered by Nobel Prize winner Daniel Kahneman (Thinking, Fast and Slow, 2011), which is based on two "systems": an intuitive System 1 which is fast, reactive, automatic, and specialized, and a reasoning System 2 which is slow, deliberative, transparent, and general. Data-based learning systems are like System 1 boxes; while model-based reasoning systems are like System 2 boxes. System 1 and System 2 processes, however, are tightly integrated in the human mind, and a main challenge is to achieve a similar integration between data-based learning and model-based reasoning in AI. Key steps in this direction and main objectives of Hector's research are learning meaningful models from data, and getting data-based and model-based components to complement, enhance, and inform each other, in the context of acting and planning.
Bio
- Hector Geffner is an Alexander von Humboldt Professor at the RWTH Aachen University, Germany, since January 2023, and a Guest Wallenberg Professor at Linköping University, Sweden, since September 2019.
- Before joining RWTH Aachen, he was an ICREA Research Professor at the Universitat Pompeu Fabra (UPF) in Barcelona, Spain, since 2001.
- Hector obtained a Ph.D. in Computer Science at UCLA in 1989 and then worked at the IBM T.J. Watson Research Center in NY, and at the Universidad Simon Bolivar in Caracas.
- Hector is a Fellow of AAAI and EurAI, and former Associate Editor of AI and JAIR.
- His most recent book, with Blai Bonet, is “A Concise Introduction to Models and Methods for Automated Planning”, Morgan and Claypool, 2013.
- His research interests are in computational models of reasoning, action, and learning that are effective and general.
- Hector received the 1990 ACM Dissertation Award and is best known for his work in planning for which he received the 2009, 2010, and 2014 ICAPS Influential Paper Awards.
- Currently, he is interested in methods for learning representations for acting and planning, and leads an ERC project in the area (RLeap, 10/2020-10/2025).
- He teaches courses on AI and on social and technological change.
- Prof. Geffner's current research is aimed at addressing the problem of learning representations from data that support reasoning, reuse, and generalization. It is a central problem in AI where deep learning yields inflexible, black boxes that cannot be trusted, and model-based approaches yield flexible and reusable behaviors but relying on handcrafted representations.
Publications
2024


2023
